Turning a corner at night, a couple of bright dots flash in front of you, standing still as your car quickly approaches them. Will you be fast enough to stop the vehicle before hitting the deer in the middle of the road?
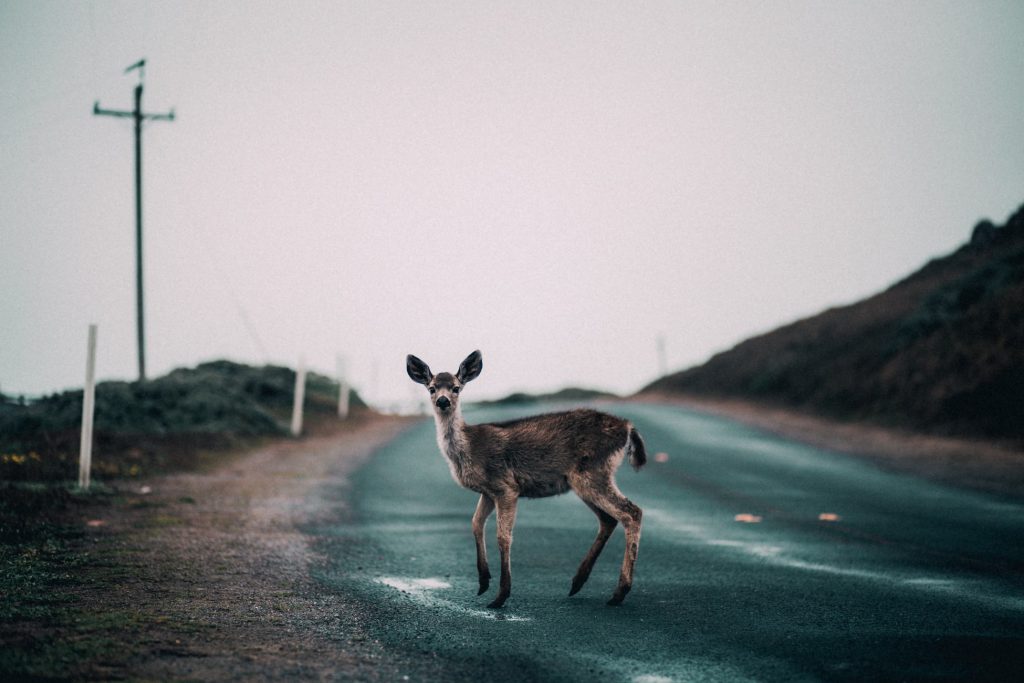
If you are used to drive in the countryside or in roads close to forests, hills, mountains, or large uninhabited regions, this scenario probably sounds familiar to you. Deer and large mammals crossing the road represent a life-threatening and common problem in many countries worldwide. In USA, it is estimated that more than a million vehicle accidents per year involve wildlife, corresponding to avove $8 billion in medical costs and car repairs annually (National Geographic, “Wildlife Crossing”).
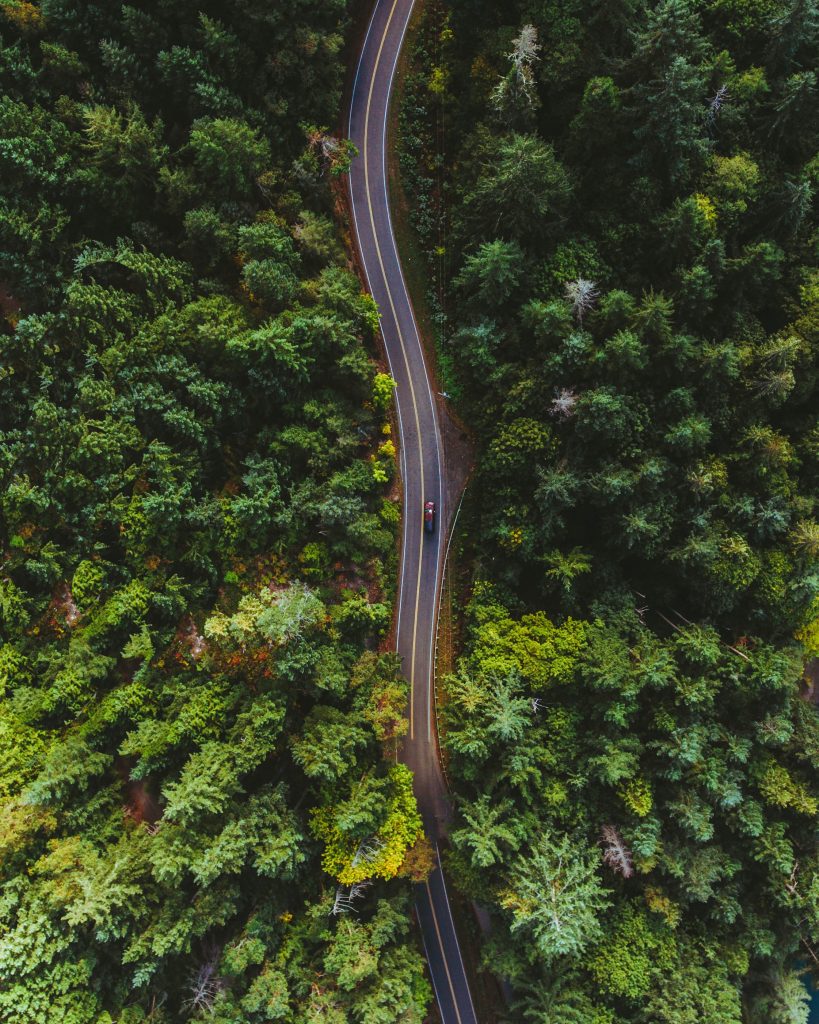
The problem is analogously relevant also from the perspective of wildlife well-being. As an example, even though formal records are only maintained in a small proportion of countries at the European level, the full toll of ungulates killed annually on European roads is estimated to be close to 1 million, with a steady increase in the last 40 years [Langbein, J., Putman, R., & Pokorny, B. (2011). Traffic collisions involving deer and other ungulates in Europe and available measures for mitigation]. Many factor contribute to the increase in Wildlife-vehicle collisions (WVC), including the development of extensive road networks affecting and isolating habitats and populations, as well as climate changes and deforestation.
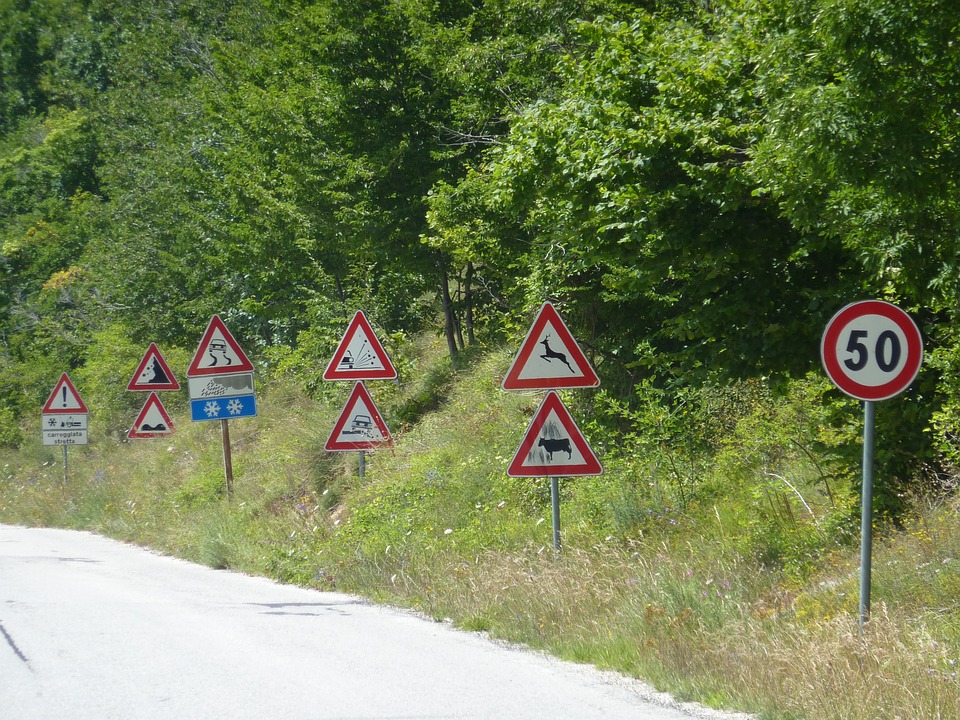
The introduction of warning signs and/or lights indicating the “potential crossing” of wildlife is a common approach to inform the drivers about the high probability of animal crossing in a certain road section. However, alarm fatigue quickly takes place especially for drivers accustomed to drive in wildlife-crossing-critical regions, with the resulting desensitization leading the warning to be practically ignored, hence making the static alarm infrastructure practically useless.
Monitoring the roadsides through video/infrared cameras to identify animals potentially crossing the road and give alerts to the driver is possible in theory. However, the cost of installing a camera every few meters, the potential visibility issues in many environmental and illumination conditions, and the cost for collecting and processing the resulting large amount of video streams in real time to provide useful warning make such a solution unpractical even for short road sections.
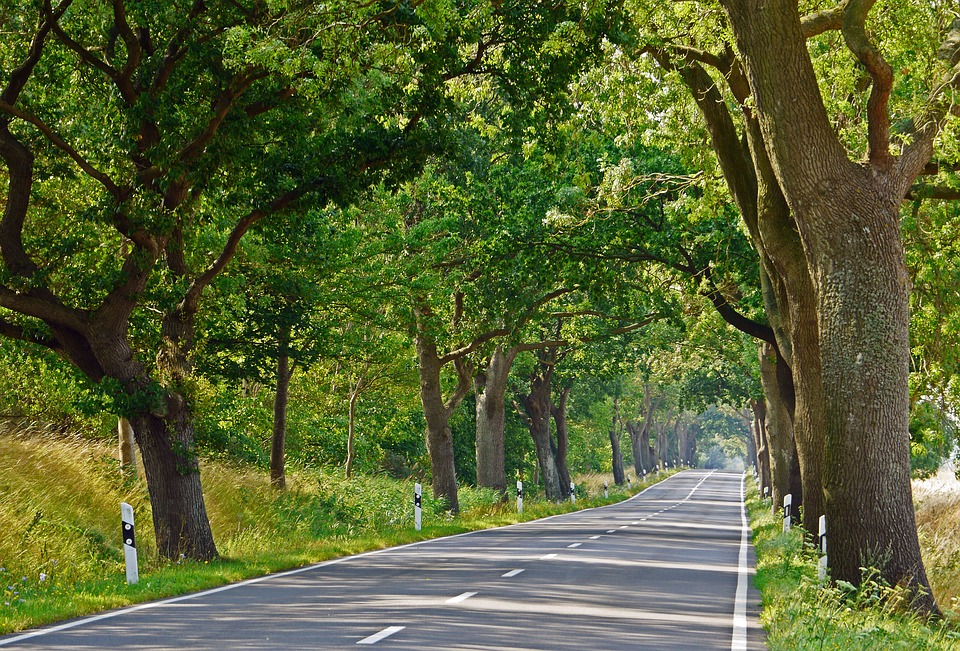
The recent advent of low-cost wireless sensing technologies has enabled a new generation of warning systems for wildlife crossing to be developed, with the ELEDIA Research Center already experimenting scalable and robust solutions within the MARGINE Project. The fundamental physical concept behind MARGINE may look trivial, since it exploits radio waves (not affected by light/visibility conditions) to detect the distance and velocity of the animals likewise any radar system would do. However, two fundamental challenges immediately arose in the path to make the system practical and useful, that is (i) how to monitor long sections of roads avoiding expensive installations, and (ii) how to enable reliable early warning (i.e., telling the driver “an animal is going to cross in 10 seconds 200 meters in front of you”).
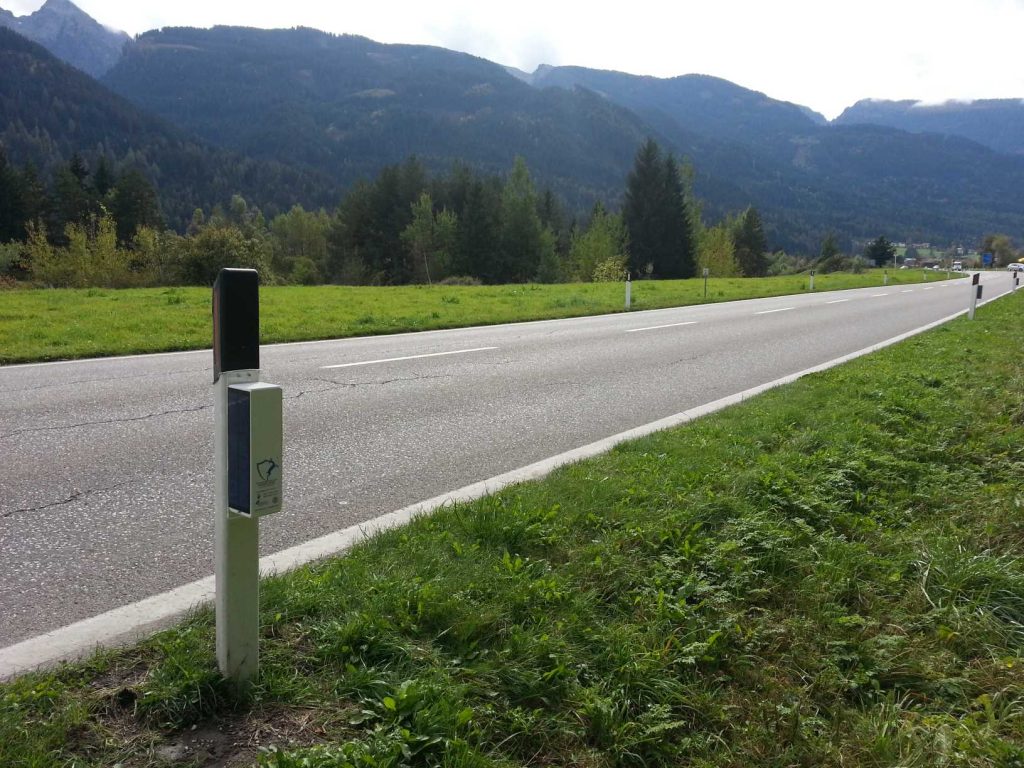
The first challenge was solved by the development and deployment of a collaborative network of wireless smart guide posts. The fundamental idea behind such a choice was to develop a relocatable guide post integrating both sensing (i.e., capability to detect of presence, speed, and distance of animals) and communications capabilities, and making the posts communicating via wireless to share the detected information to far-away sections of the road in a matter of milliseconds (more detail on the technological aspects can be found in the project page). Yet the second issue cannot be solved by sensing alone, as it is actually related more to understanding the animal behavior than to just detecting it. That is where the AI methodologies developed by the ELEDIA Research Center made the difference.
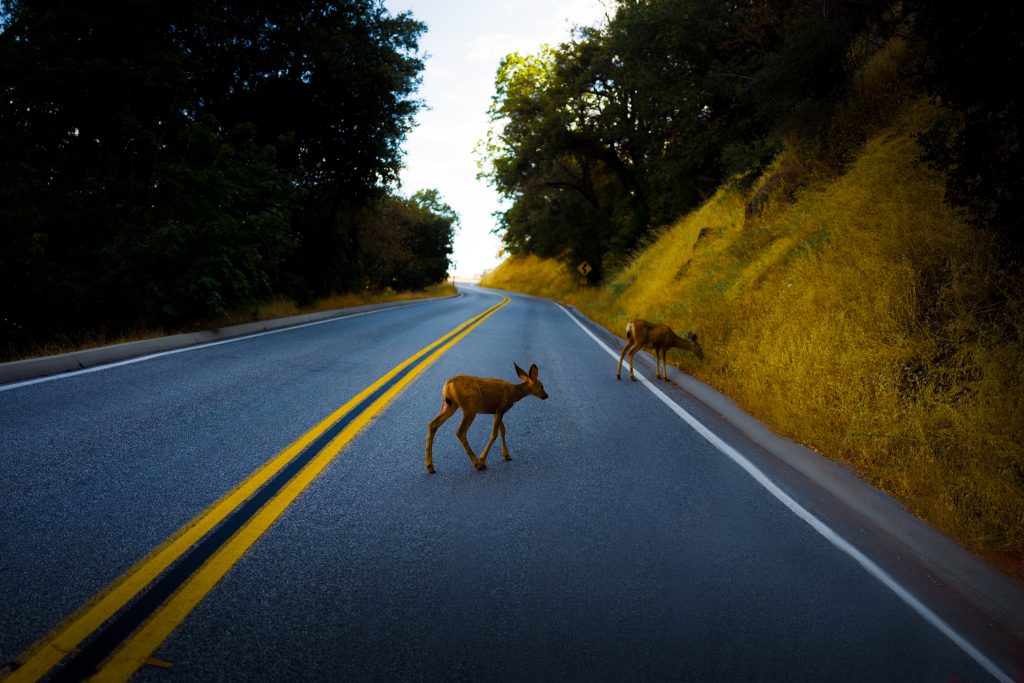
Each wild animal has a different path of movement, which can be studied to understand important migration information [D. R. Rubenstein, K. A. Hobson, “From birds to butterflies: animal movement patterns and stable isotopes,” Trends in Ecology & Evolution, vol. 19, no. 5, pp. 256-263, 2004.] On a smaller scale, ungulates crossing a road exhibit a very specific behavior, where the animal go through a set of different phases (such as “study”, “decision”, “crossing”) that can be identified to predict their subsequent action and likelihood to enter a dangerous condition. Understanding the behavioral patterns of large mammals (such as deers and wild boars) when reaching a roadside has been the fundamental challenge addressed by the E-AIR methodological suite within MARGINE. Thanks to the experimental data collected in collaboration with the Autonomous Province of Trento and the Associazione Cacciatori Trentini, the members of the ELEDIA Research Center were able to develop and validate an AI-powered behavioural model for wildlife crossing. Such a model has been implemented, calibrated, and experimentally demonstrated in the MARGINE Test Sites, located in Cavalese, Predazzo, and Ziano di Fiemme, Val di Fiemme, Trento (Italy).
In the deployed MARGINE system, the data collected by the smart guide posts are gathered and processed in a central unit (the “MARGINE Hub”). By exploiting the developed ELEDIA artificial intelligence suite, the MARGINE Hub infers the presence of animals and their behavior when approaching the road, then presenting a warning through a variable message sign only if a potentially dangerous condition is detected. To achieve this goal, a combination of machine learning, evolutionary optimization, deep-learning, and fuzzy-logic techniques have been implemented, leading to a general approach that can be easily adopted regardless of the road features and sensing architecture (number and position of sensors, distance, implemented technology). Beyond the obvious safety benefits and increased infrastructure support to road managers, such a solution enables to minimize the warnings to drivers and avoid the arising “alarm fatigue”. Moreover, it can be easily customized to handle different classes of animals including bears, elks, moose, kangaroos, and bison. For instance, the detection of wild boar has been already validated by ELEDIA in the Parco Colli Euganei, Padova),
So, saving Bambi is always about understanding it, after all.
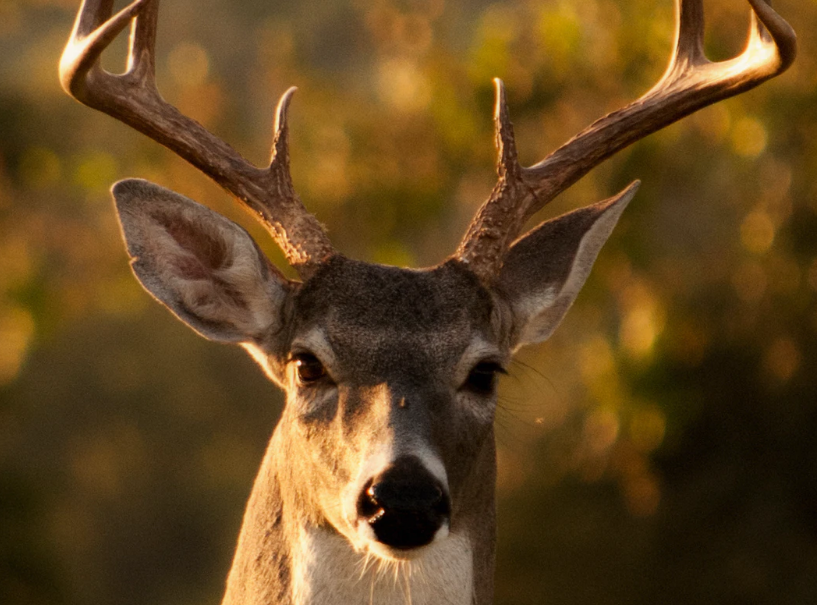
Read more
- The MARGINE Project http://www.eledia.org/showcase/margine/ (in Italian)
- G. Beltrami, “Sensori sulla strada per salvare gli animali”, L’Adige newspaper, published 10/07/2020 (in Italian)
- “Nuovi sensori stradali avvisano della presenza di animali”, L’Adige.it, published 05/11/2014 (in Italian)
- L. Piva, “Un dispositivo wireless per rilevare la presenza di cinghiali”, Il Gazzettino newspaper, published 07/01/2018 (in Italian)
- F. Viani, F. Robol, M. Salucci, E. Giarola, S. De Vigili, M. Rocca, F. Boldrini, G. Benedetti, and A. Massa, “WSN-based early alert system for preventing wildlife-vehicle collisions in Alps regions – From the laboratory test to the real-world implementation,” EuCAP 2013, Gothenburg, Sweden, pp. 1857-1860, April 8-12, 2013.
- F. Viani, F. Robol, E. Giarola, G. Benedetti, S. Devigili, and A. Massa, “Advances in wildlife road-crossing early-alert system: new architecture and experimental validation”, 8th European Conference on Antennas and Propagation (EUCAP 2014), The Hague, The Netherlands, pp. 3457-3461, April 6-11, 2014.
- F. Viani, F. Robol, A. Polo, and E. Giarola, “Wildlife road-crossing monitoring system: Advances and test site validation,” 10th European Conference on Antennas and Propagation (EUCAP 2016), Davos, Switzerland, pp. 1-4, April 11-15, 2016.
- F. Viani, A. Polo, E. Giarola, G. Benedetti, S. Zanetti, and F. Robol, “Performance assessment of a smart road management system for the wireless detection of wildlife road-crossing,” 2016 IEEE International Smart Cities Conference (ISC2), Trento, Italy, pp. 1-6, September 12-15, 2016.